|
楼主 |
发表于 2023-12-1 10:59:07
|
显示全部楼层
UNRAVELING THE MYSTERY OF DRUG SPECIFICITY: THE CASE OF BLEBBISTATIN
November 30, 2023
by Greg Bowman
When it comes to designing novel drugs, achieving specificity is a major challenge. An effective drug must bind tightly to its target protein while avoiding unwanted side effects that can result from interactions with other proteins. This challenge becomes even more complex when targeting specific members of protein families with similar structures. Additionally, some enzymes share substrates, like ATP, across various protein families, making it difficult to design compounds that compete with endogenous ligands without causing off-target effects.
One innovative approach to drug design is targeting allosteric sites rather than active sites. Allosteric compounds can enhance desirable protein functions, offering a unique way to achieve specificity. These sites are often less conserved than active sites, making it easier to develop specific drugs. In recent years, highly specific allosteric compounds have been serendipitously discovered through high-throughput screens, targeting various proteins such as G-protein-coupled receptors, myosins, kinases, and β-lactamases. Despite these successes, designing drugs that target allosteric sites from scratch is challenging because experimental structural studies often provide limited insights into a protein’s conformational landscape.
One specific area of interest is myosins, a superfamily of ATPases that play crucial roles in various cellular processes. Myosins have the potential to be valuable drug targets for numerous diseases, but their complexity and the existence of multiple isoforms make targeting specific myosin variants extremely difficult. For instance, there are 38 myosin genes in the human genome, and individual cells express about 20 different myosin isoforms. Compounds like mavacamten have shown promise in clinical trials for heart-related conditions, but there is a need for more myosin modulators to address a broader range of diseases. However, the challenge lies in targeting specific myosin isoforms due to their highly conserved motor domain fold and active site structure.
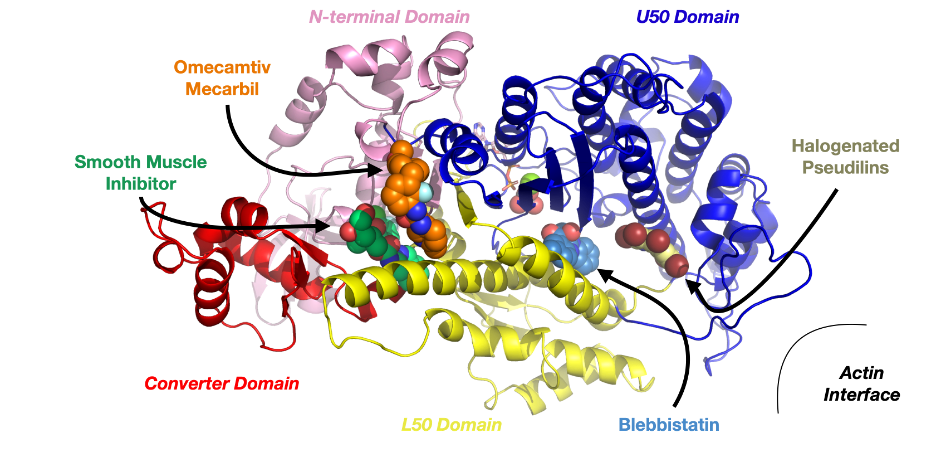
Figure caption: Structure of a myosin protein highlighting the binding sites of some known allosteric modulators, including blebbistatin.
Blebbistatin, a myosin-II specific allosteric inhibitor, has been a subject of study to understand the molecular mechanisms governing drug specificity. It was discovered in a high-throughput screen targeting nonmuscle myosin IIs and was found to broadly inhibit various myosin-II isoforms while sparing other myosin families. The key to its selectivity lies in the dynamics of the blebbistatin pocket and the conformations myosin isoforms adopt in solution.
Through all-atom molecular dynamics simulations, this study has shown that the probability of the blebbistatin pocket opening is higher in more sensitive myosin isoforms, which explains differences in drug potency. This finding, along with differences in the pocket’s residue composition, provides insights into the factors contributing to drug specificity. These results demonstrate the role of pocket dynamics and conformational selection in achieving drug specificity and highlight the potential for precision medicine through computational modeling.
In conclusion, the study of blebbistatin sheds light on the intricate world of drug specificity in the realm of myosin inhibitors. It emphasizes the importance of understanding the dynamic interplay between drug molecules and protein structures. This knowledge has the potential to open doors to more precise drug design, allowing us to target specific isoforms and improve the effectiveness of therapeutic interventions. As the field of precision medicine advances, computational modeling and simulations like the ones used in this study offer promising opportunities to tailor treatments to individual patients and address a wide range of diseases with unprecedented specificity.
WCG Team
译文:
揭开药物特异性之谜:白比他汀案例
2023年11月30日
作者 格雷格·鲍曼
在设计新型药物时,实现特异性是一个重大挑战。一种有效的药物必须与它的目标蛋白紧密结合,同时避免与其他蛋白相互作用,以免可能产生的不必要的副作用。当针对具有类似结构的蛋白质家庭的特定成员时,这一挑战就变得更加复杂。此外,一些酶在不同的蛋白家族中具有像三磷酸腺苷一样的底物,因此很难设计出能与内源性配体竞争而又不会产生目标外效应的化合物。
一个创新的药物设计方法是针对异形部位而不是活跃部位。异体化合物能增强理想的蛋白质功能,为实现特异性提供了独特的途径。这些异形部位往往比活跃部位的更不保守,因此更容易开发出特定的药物。近年来,高特异性的异体化合物通过高通量筛选偶然被发现,其目标是各种蛋白质,如G蛋白耦合受体、肌蛋白、激酶和β-内酰胺酶。尽管取得了这些成功,但是从零开始设计针对异体部位的药物是很有挑战性的,因为实验结构研究通常对蛋白质的构型提供的见解有限。
一个特殊的关注领域是肌蛋白,一个在各种细胞过程中起关键作用的总科。肌球蛋白有可能成为许多疾病的重要药物靶点,但它们的复杂性和多种异形体的存在使针对特定的肌球蛋白变异体的靶向极其困难。例如,人类基因组中有38个肌球蛋白基因,单个细胞表达大约20个不同的肌球蛋白异型。在心脏病的临床试验中,像Mavacamten这样的化合物已经显示出了希望,但是需要更多的肌球蛋白调节剂来治疗更广泛的疾病。然而,由于其高度保守的运动域折叠和活跃的部位结构,目前的挑战在于如何定位特定的肌球蛋白异形。
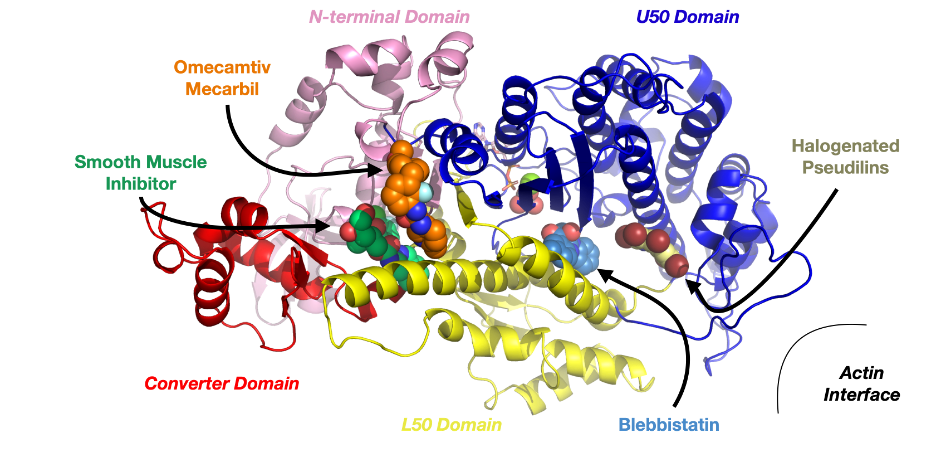
图说明:肌球蛋白的结构,突显了一些已知的异体调节器的结合部位,包括布比他汀。
一种II型肌蛋白的特异性异体体抑制剂布利比他汀一直是研究的课题,以了解控制药物特异性的分子机制。在一个针对II型非肌肌球蛋白的高通量筛选中发现了它,并发现它广泛抑制各种II型肌蛋白异型,同时绕开了其他肌蛋白家族。选择性的关键在于布比司他汀口袋的动力学和溶液中采用的肌蛋白异型。
通过全原子分子动力学模拟, 这项研究结果表明,在更敏感的肌球蛋白异形体中,布比他汀口袋开口的概率更高,这解释了药物效力的差异。这个发现,以及口袋里残基成分的差异,提供了对药物特异性的因素的深入了解。这些结果说明了口袋动力学和构象选择在实现药物特异性方面的作用,并通过计算建模强调了精密医学的潜力。
总之,对布比他汀的研究揭示了肌球蛋白抑制剂领域药物特异性的复杂世界。它强调了理解药物分子和蛋白质结构之间动态相互作用的重要性。这种知识有可能为更精确的药物设计打开大门,使我们能够针对特定的异形体设计药物,并提高治疗干预的有效性。随着精密医学领域的发展,像本研究中所使用的那样的计算建模和模拟为针对个别患者的治疗提供了有希望的机会,并以前所未有的特异性来治疗广泛的疾病。 |
|